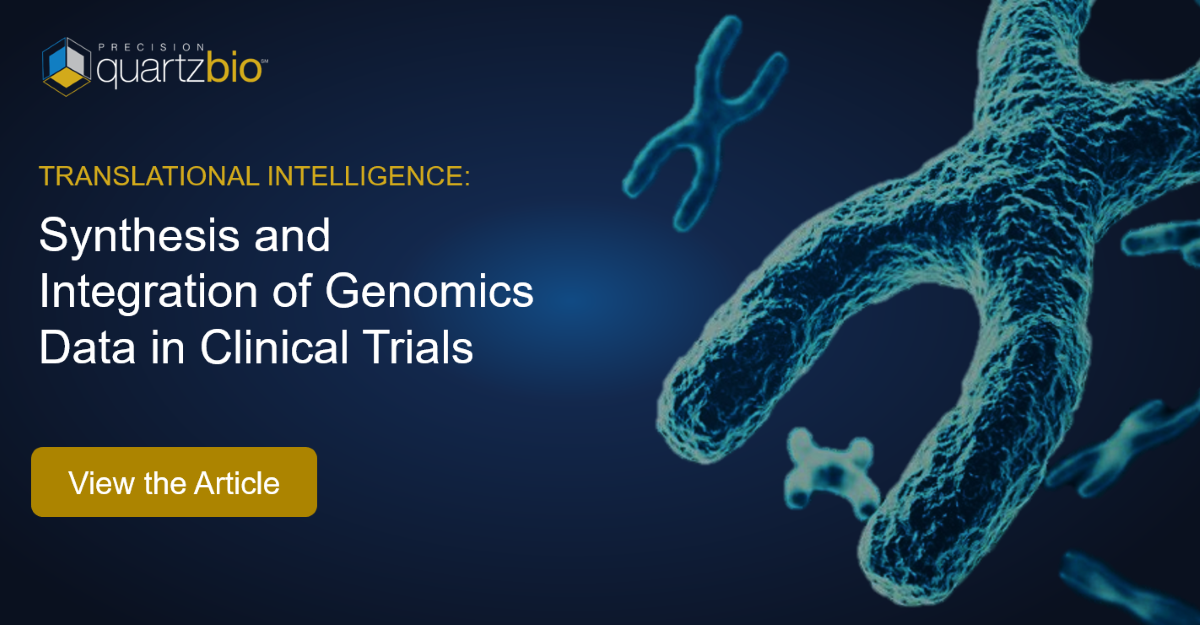
April 21, 2021 — A modern clinical trial, with well-characterized subjects studied over periods of time, presents unmissable opportunities for sponsors to characterize mechanism of action, prioritize target pathways for their pipelines, and generate as much data as possible to support regulatory filings.
For maximum insight generation, sponsor teams must have the ability to flexibly interrogate the breadth and depth of their clinical and biomarker assay data. We have discussed aspects of this need in prior pieces, covering integration across clinical, PK, and exploratory data, as well as the challenges that arise due to inconsistent data standards.
Both the breadth and depth of data generated in clinical trials are growing. For example, we see that genomics and transcriptomics are now part of most translational data packages – and these data sets are far from one-dimensional. Given the incredible potential of genomics and gene expression profiling for patient selection and signature development (Figure 1), sponsors have many incentives to optimize efficient interrogation of this multi-dimensional data.
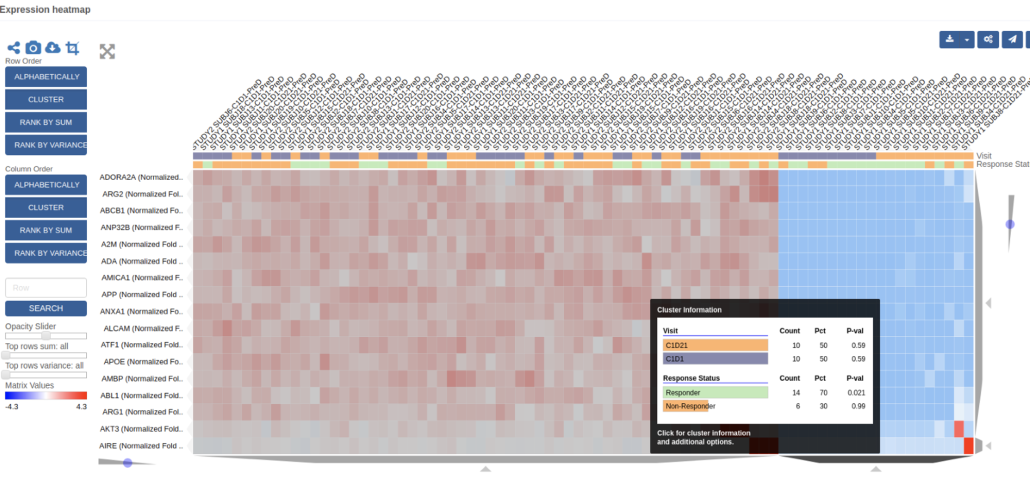
Figure 1. Depth of genomic and transcriptomic profiling now commonly enables high dimensional analyses. Gene expression linked to clinical metadata enables signature development. Unsupervised clustering of this data allows for quick correlation between the metadata, such as response status.
Linking these data to clinical metadata and outcomes is an extraordinary opportunity to rapidly generate and prioritize hypotheses, going much deeper than would be possible through targeted/directed analyses alone. However, generating a hypothesis is only the beginning.
Immediate next steps to investigating a hypothesis based on genomics and gene expression data include:
- Check quality control (QC) parameters of assay data.
- Evaluate pre-analytical variables, such as RNA / DNA yield, viability, and processing time.
- Determine what pathways are implicated by the genes identified in the hypothesis. Are genes in those pathways differentially expressed with respect to patient subpopulation?
- Determine what published findings in the literature are connected to these genes. Interrogate public databases, such as the GWAS catalog from NCBI, or gnomAD annotations.
Today, we highlight the power of linking “reportables” to ancillary files (e.g. variant call format (VCF) files, PDFs) as well as the value of seamlessly connecting to contextual information (e.g. variant databases and gene network maps). This ability to rapidly assess underlying data and connect to broader data sets is often the difference between finding correlation in large scale data sets and achieving “Translational Intelligence” (which we define as insights that help prioritize the most productive areas to focus).
To perform any of the above queries, genomic and transcriptomic data must first be ingested and connected to sample data, assay metadata, clinical data as well as knowledgebases and public datasets / databases.
However, ingestion and connection of genomics data at scale can be challenging. There is a huge variety of the structure and format of genomic and gene expression data, ranging from Variant Call Format (VCF) files to Reporter Code Count (.RCC) files (Figure 2). In fact, common genomic profiling services continue to deliver key information within PDFs.
![]() |
![]() |
![]() |
![]() |
VCF | DNA-seq (FastQ) | RCC |
Figure 2. Examples of Genomics and Gene Expression Assay Data Delivered in Diverse Formats, High-dimensional Data Structure
Genomics Module Connects Clinical Trial Data With Third-Party Information
To enable efficient exploration of genomic and transcriptomic data in clinical trials, QuartzBio recently expanded its genomics module as part of its biomarker data management solution – the current module is linked to multiple external databases and third-party applications to provide robust contextual information at the click of a mouse.
Among other capabilities, the genomics module integrates VCF file data to enable interactive exploration of genomic context for mutation calls. The expanded genomics module also allows for data generated during clinical trials to be interrogated using third-party tools, such as the gnomAD annotation database and the GeneMANIA network mapping tool (Figure 3). Translational research teams can use this module to investigate the functional consequence of genes identified during signature exploration, within the context of known gene function(s) upstream and downstream in pathway(s) of interest.
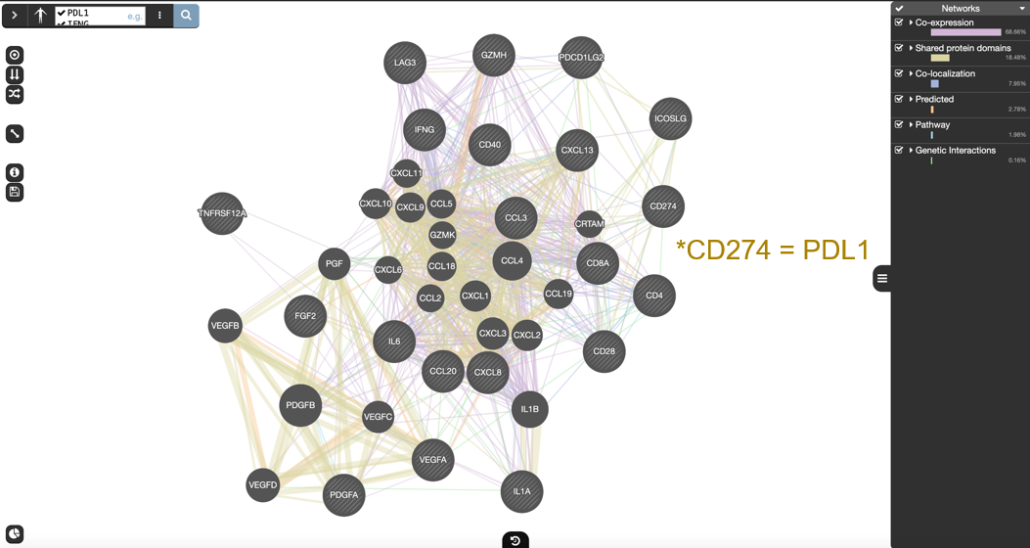
Figure 3. Explore and query pathways and networks of interest in your clinical trial data with deeper context. In this example shown, investigate PDL1 as a biomarker of immuno-oncology response and what pathways are active up- and downstream, using the GeneMania network mapping tool within the QuartzBio platform.
Is your team tackling the challenges and opportunities of genomic data generated during clinical trials?
Join us for a webinar where Tobi Guennel at QuartzBio will present a case study on how teams are quickly identifying genes of interest and evaluating IO response signatures in their clinical trial genomic data.