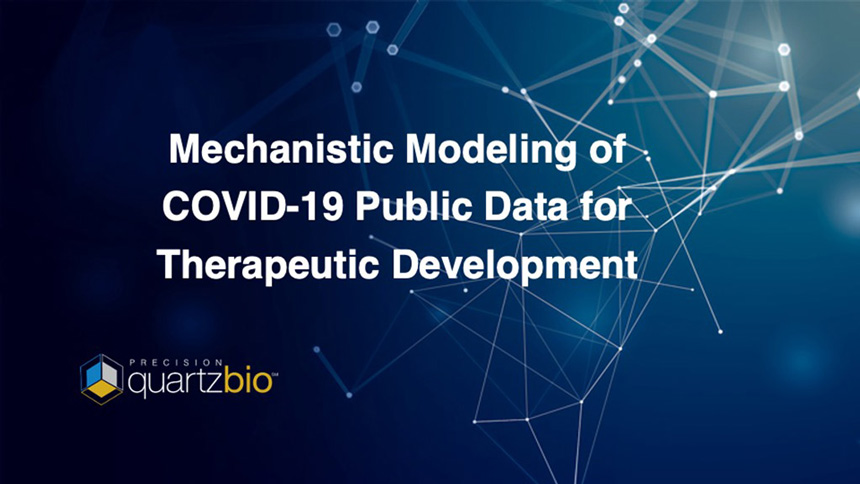
June 8, 2020 — As the QuartzBio team’s efforts have progressed with the selection and integration of SARS-CoV-2 / COVID-19 data sets, I am pleased to share our first public update on analysis. This work builds on an evaluation of IFN-α2b treatment, and supports the rationale that interferons are upregulated as part of the antiviral defense, specifically for COVID-19. We are also sharing this work as a demonstration of the potential for translational research and drug repurposing while mitigating the time, expense, and reproducibility challenges inherent in drug/treatment combination discovery. This need for speed and flexibility is, of course, at the top of our minds as we respond to the global pandemic.
As the QuartzBio team’s efforts have progressed with the selection and integration of SARS-CoV-2 / COVID-19 data sets, I am pleased to share our first public update on analysis. This work builds on an evaluation of IFN-α2b treatment, and supports the rationale that interferons are upregulated as part of the antiviral defense, specifically for COVID-19. We are also sharing this work as a demonstration of the potential for translational research and drug repurposing while mitigating the time, expense, and reproducibility challenges inherent in drug/treatment combination discovery. This need for speed and flexibility is, of course, at the top of our minds as we respond to the global pandemic.
Our team interrogated SARS-CoV-2 / COVID-19 RNAseq data kindly published by Melo-Blanco et al., at the Icahn Institute of medicine at Mt. Sinai (GSE147507) using a causal mechanistic modeling approach. Enabling this was a computable graph knowledge base that enables systematic pattern matching between transcriptional profile of SARS-CoV-2 infection and the transcriptional patterns of thousands of other experiments and studies. Among other mechanisms, upregulation of IFN-α family signaling (i.e., a gene expression pattern consistent with increased activation of the IFN-α pathway, Figure 1) was predicted in normal human bronchial epithelial cells infected with SARS-CoV-2, as well as in a COVID-19 patient that succumbed to the disease. This supports the rationale that an interferon program is activated as an anti-viral defense, specifically for COVID19, a finding that was also observed by the authors of the published experiment.
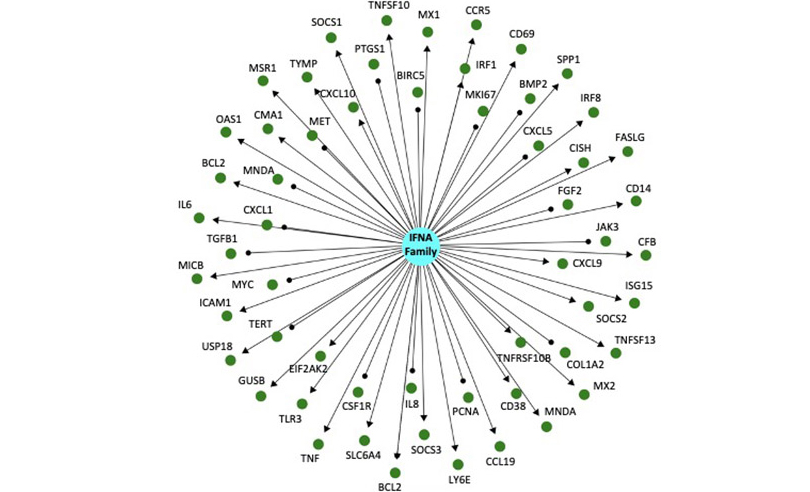
Figure 1: This is a directed knowledge graph depicting mRNA expression downstream of interferon alpha. The IFNA family of proteins is represented by the blue circle in the center, and the green circles surrounding it represent indirect downstream mRNAs targets. The graph is directed, which means that the relationship between IFNA family signaling and the mRNA is known. Arrows indicate a positive regulatory relationship (increased IFNA signaling causes increased mRNA expression) or a negative regulatory relationship (increased IFNA signaling causes decreased mRNA expression). Significant changes in expression after infection are identified, and mapped on to the network above and thousands others like it. Statistical tests are performed to determine enrichment (is IFNA signaling induced after infection?) and directional consistency (is IFNA signaling increased or decreased after infection?).
These findings also provide a mechanistic rationale for interferon treatment as a potential COVID-19 therapeutic; Type 1 interferons (IFN-α, IFN-β) are well characterized primary inflammatory effectors after infection, and interferon treatments are gaining attention as a potential therapy for COVID-19 (reviewed here by Sallard et al.). Notably, there is a trial underway exploring IFN-β treatment for MERS-CoV, and a recent publication in Frontiers in Immunology describes an exploratory study where COVID-19 patients were treated with interferon-α2b with the following outcome: “Treatment with IFN-α2b with or without arbidol (the broad-acting anti-viral, Umifenovir) significantly reduced the duration of detectable virus in the upper respiratory tract and in parallel reduced duration of elevated blood levels for the inflammatory markers IL-6 and CRP”.
Even with clinical trials being planned or underway, there is always room to investigate the mechanistic rationale behind a repurposed therapy for a new condition using pre-clinical and computational models. While mice are the most ubiquitous animal model for drug research, they are not particularly durable hosts for SARS-CoV-2 replication (see here for a discussion in Science about finding the right animal model for COVID-19 research). In fact, the Melo-Blanco et al. data set, included results from experiments done in ferrets (!). In order to integrate these data with data collected from humans, we’ve mapped the ferret genome to human orthologs, and are beginning our analysis into the translatability of this model.
In recent years, common approaches to explore translational interferon research would likely have required near exclusive use of animal or in vitro models, requiring months of work to complete. Recognizing the urgency of combatting the global pandemic, we believe this is an especially important time to acknowledge Mt Sinai’s contribution in making this data available to support broader research and development. We further believe this is an opportune moment of our industry to consider computational and mechanistic methodologies to advance insight generation given the following rationale:
- With a data asset already integrated as part of the COVID-19 data aggregation initiative, there is significant opportunity to compress timelines and extend potential pathways for analysis. For example, teams can work directly with our integrated COVID-19 data assets and complete the above analysis in a matter of days. We often work with our partners to do exactly this.
- We also appreciate that animal models do not consistently recapitulate human physiology and disease biology. We often apply computational approaches to mechanistically model the similarities and differences between the preclinical model and the clinic, and overlay the MOA to ensure it lies in the intersection. Put another way, we want to make high resolution, data-driven decisions about pre-clinical model select, and once chosen, understand what it can and cannot be used for.
This analysis is a small window into what we hope the work we’re completing with our COVID-19 data integration initiative will enable to advance COVID-19 research. We will provide updates in coming weeks as we extend to molecular signatures of drug treatment from resources like LINCS, and patient data from COVID-19 relevant diseases like Kawasaki Disease and or rheumatic conditions (e.g., lupus). We particularly welcome your feedback as we prepare for a more formal update on our work via a webinar in coming weeks – and please share if there is anything we can provide to help advance your work.
Author: Renée Deehan | VP, Computational Biology at QuartzBio, part of Precision for Medicine